NLP 中的对比学习
对比学习的目标是学习这样的嵌入空间,其中相似的样本彼此靠近而不同的样本相距很远。它假设一组成对的句子,例如 ,其中 xi 和 xi+ 在语义上彼此相关。
让和
表示 x_i 和 {
,对于具有 N 对的小批量,训练目标为
是:
其中 \tau 是温度超参数,而 sim( ) 表示编码输出的余弦相似度,可以使用预训练的语言模型(如 BERT 和 RoBERTa)生成
它可以应用于有监督和无监督的设置。在本文中,我们将讨论对比学习的一个特定领域,即文本增强。
文本增强:
计算机视觉中的对比学习只是生成图像的增强。构建文本增强比图像增强更具挑战性,因为我们需要保留句子的含义。有 3 种增强文本序列的方法:
回译
在这种方法中,我们尝试通过反向翻译生成增强句子。 CERT(来自变压器的对比自监督编码器表示)就是这样一篇论文。在本文中,许多不同语言的翻译模型用于输入文本的文本增强,然后我们使用这些增强来生成文本样本,我们可以使用对比学习的不同记忆库框架来生成句子嵌入。
词法编辑:
词法编辑或 EDA(简易数据增强)是一组简单的文本增强操作。它需要一个句子作为输入并随机应用四个简单操作之一:
- 随机插入(RI):在句子中随机位置插入随机选择的非停用词的同义词。
- 随机交换 (RS):将两个单词随机交换 n 次。
- 随机删除(RD):以概率 p 随机删除句子中的每个单词。
- 同义词替换 (SR): 从句子中随机选择 n 个非停用词。用随机选择的同义词之一替换这些单词中的每一个。
虽然这些方法简单、易于实现,但作者也承认存在局限性,即它们在小数据集上的改进小于 1%,而在用作预训练模型时几乎没有改进。
截止和辍学:
隔断:
2009 年,微软和伊利诺伊大学的 Shen 等人提出了一种使用 cutoff 进行文本增强的方法。他们提出了 3 种不同的截止增强策略。我们将一一讨论这些策略,但让我们考虑将句子表示为 L*d 的嵌入,其中 L 是特征的数量,d 是句子的长度
- 特征截断:删除一些选定的特征。
- Token Cutoff:移除少数选中的Token的信息。
- 跨度截止:删除连续的文本块。
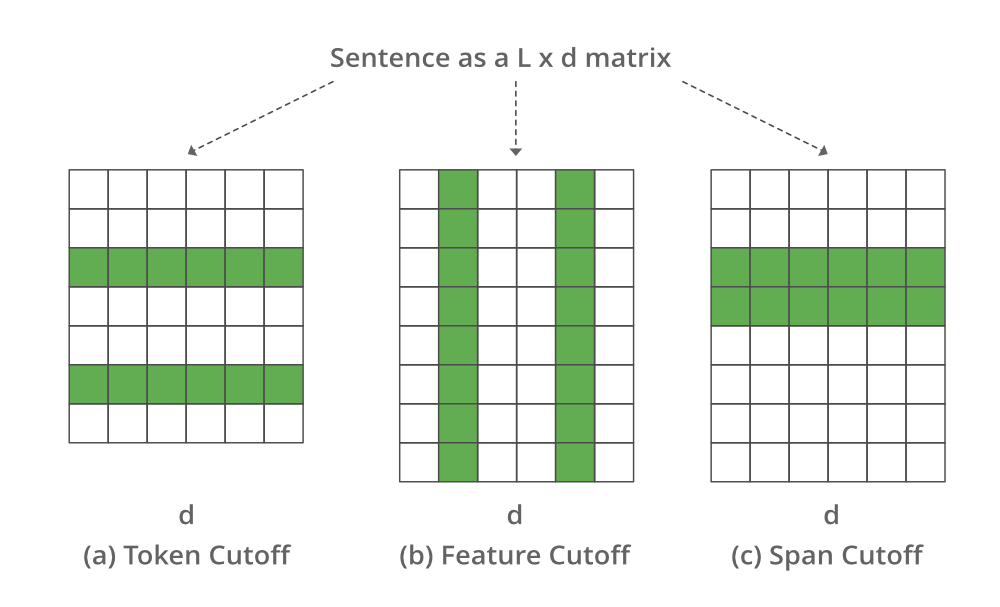
代表不同截止值的图像
为了比较来自不同增强的预测分布,我们在训练期间使用了额外的 KL 散度损失。
退出:
2021 年,斯坦福 NLP 小组的研究人员提出了 SimCSE。 在无监督场景中,它学习使用 DropOut 噪声预测句子本身,换句话说,他们将 dropout 视为文本序列的数据增强。
在上述方法中,我们取一组句子 {[x_i ]}_{i=1}^{m} 并将正对视为自身 x_i^{+} = x_i。在 Transformer 的训练过程中,有一个 dropout 掩码和注意力概率应用于全连接层。它通过应用不同的 dropout mask z, z 0简单地将相同的输入两次馈送到编码器,因此训练目标函数可以定义为:
其中,h_i^{z_i} = f_\theta (x_i, z) 是输出,z 是 dropout 的标准掩码。
在监督设置中,我们尝试利用自然语言推理数据集 (NLI) 来预测给定的句子对之间是否存在蕴涵(正对)或矛盾(负对)。我们延长目标可以定义为:
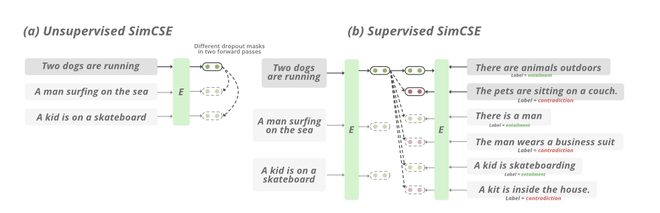
SimCSE的工作
执行
- 在这个实现中,我们取几个句子,然后应用 SimCSE 标记器对句子和模型进行标记以生成输入的嵌入,然后我们计算它们的余弦相似度。
Python3
# Install PyTorch
! pip install torch==1.7.1+cu110 -f https://download.pytorch.org/whl/torch_stable.html
# clone the repo
!git clone https://github.com/princeton-nlp/SimCSE
# install the requirements
! pip install -r /content/SimCSE/requirements.txt
# imports
import torch
from scipy.spatial.distance import cosine
from transformers import AutoModel, AutoTokenizer
# Import the pretrained models and tokenizer, this will also download and import th
tokenizer = AutoTokenizer.from_pretrained("princeton-nlp/sup-simcse-bert-base-uncased")
model = AutoModel.from_pretrained("princeton-nlp/sup-simcse-bert-base-uncased")
# input sentence
texts = [
"I am writing an article",
"Writing an article on Machine learning",
"I am not writing.",
"the article on machine learning is already written"
]
# tokenize the input
inputs = tokenizer(texts, padding=True, truncation=True, return_tensors="pt")
# generate the embeddings
with torch.no_grad():
embeddings = model(**inputs, output_hidden_states=True,
return_dict=True).pooler_output
# the shape of embedding (# input, 768)
embeddings.shape
# print cosine similarity b/w the sentences
for i in range(len(texts)):
for j in range(len(texts)):
if (i != j):
cosine_sim = 1 - cosine(embeddings[i], embeddings[j])
print("Cosine similarity between \"%s\" and \"%s\" is: %.3f" % (texts[i], texts[j], cosine_sim))
Cosine similarity between “I am writing an article” and “Writing an article on Machine learning” is: 0.515
Cosine similarity between “I am writing an article” and “I am not writing.” is: 0.517
Cosine similarity between “I am writing an article” and “the article on machine learning is already written” is: 0.441
Cosine similarity between “Writing an article on Machine learning” and “I am writing an article” is: 0.515
Cosine similarity between “Writing an article on Machine learning” and “I am not writing.” is: 0.188
Cosine similarity between “Writing an article on Machine learning” and “the article on machine learning is already written” is: 0.807
Cosine similarity between “I am not writing.” and “I am writing an article” is: 0.517
Cosine similarity between “I am not writing.” and “Writing an article on Machine learning” is: 0.188
Cosine similarity between “I am not writing.” and “the article on machine learning is already written” is: 0.141
Cosine similarity between “the article on machine learning is already written” and “I am writing an article” is: 0.441
Cosine similarity between “the article on machine learning is already written” and “Writing an article on Machine learning” is: 0.807
Cosine similarity between “the article on machine learning is already written” and “I am not writing.” is: 0.141
- 在上面的例子中,我们只是比较了句子的余弦相似度。如需了解更多培训详情,请查看官方仓库中的培训详情。
参考:
- SimCSE 论文
- CERT论文
- 截断文本增强
- L'il Wong 博客