梯度下降的矢量化
在机器学习中,回归问题可以通过以下方式解决:
1. 使用优化算法——梯度下降
- 批量梯度下降。
- 随机梯度下降。
- 小批量梯度下降
- 其他高级优化算法,如(共轭下降……)
2. 使用正态方程:
- 使用线性代数的概念。
让我们考虑单变量线性回归问题的批量梯度下降的情况。
这个回归问题的成本函数是:
目标:

为了解决这个问题,我们可以采用矢量化方法(使用线性代数的概念)或非矢量化方法(使用 for 循环)。
1. 非向量化方法:
这里为了解决下面提到的数学表达式,我们使用for 循环。
The above mathematical expression is a part of Cost Function.
The above Mathematical Expression is the hypothesis.
代码:Unvectorzed Grad 的Python实现
# Import required modules.
from sklearn.datasets import make_regression
import matplotlib.pyplot as plt
import numpy as np
import time
# Create and plot the data set.
x, y = make_regression(n_samples = 100, n_features = 1,
n_informative = 1, noise = 10, random_state = 42)
plt.scatter(x, y, c = 'red')
plt.xlabel('Feature')
plt.ylabel('Target_Variable')
plt.title('Training Data')
plt.show()
# Convert y from 1d to 2d array.
y = y.reshape(100, 1)
# Number of Iterations for Gradient Descent
num_iter = 1000
# Learning Rate
alpha = 0.01
# Number of Training samples.
m = len(x)
# Initializing Theta.
theta = np.zeros((2, 1),dtype = float)
# Variables
t0 = t1 = 0
Grad0 = Grad1 = 0
# Batch Gradient Descent.
start_time = time.time()
for i in range(num_iter):
# To find Gradient 0.
for j in range(m):
Grad0 = Grad0 + (theta[0] + theta[1] * x[j]) - (y[j])
# To find Gradient 1.
for k in range(m):
Grad1 = Grad1 + ((theta[0] + theta[1] * x[k]) - (y[k])) * x[k]
t0 = theta[0] - (alpha * (1/m) * Grad0)
t1 = theta[1] - (alpha * (1/m) * Grad1)
theta[0] = t0
theta[1] = t1
Grad0 = Grad1 = 0
# Print the model parameters.
print('model parameters:',theta,sep = '\n')
# Print Time Take for Gradient Descent to Run.
print('Time Taken For Gradient Descent in Sec:',time.time()- start_time)
# Prediction on the same training set.
h = []
for i in range(m):
h.append(theta[0] + theta[1] * x[i])
# Plot the output.
plt.plot(x,h)
plt.scatter(x,y,c = 'red')
plt.xlabel('Feature')
plt.ylabel('Target_Variable')
plt.title('Output')
输出:
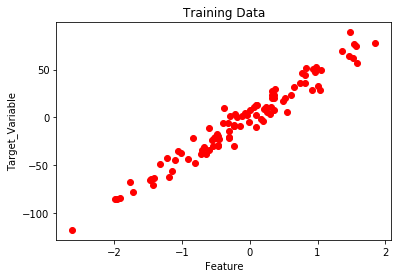
model parameters:
[[ 1.15857049]
[44.42210912]]
Time Taken For Gradient Descent in Sec: 2.482538938522339
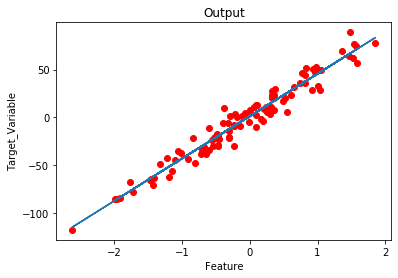
2.矢量化方法:
这里为了解决下面提到的数学表达式,我们使用矩阵和向量(线性代数)。
The above mathematical expression is a part of Cost Function.
The above Mathematical Expression is the hypothesis.
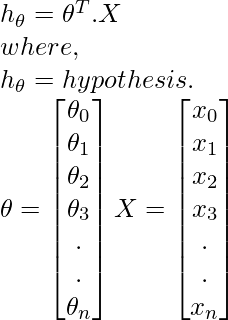
批量梯度下降:
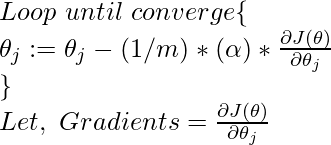
使用矩阵运算查找梯度的概念:
代码:矢量化梯度下降方法的Python实现
# Import required modules.
from sklearn.datasets import make_regression
import matplotlib.pyplot as plt
import numpy as np
import time
# Create and plot the data set.
x, y = make_regression(n_samples = 100, n_features = 1,
n_informative = 1, noise = 10, random_state = 42)
plt.scatter(x, y, c = 'red')
plt.xlabel('Feature')
plt.ylabel('Target_Variable')
plt.title('Training Data')
plt.show()
# Adding x0=1 column to x array.
X_New = np.array([np.ones(len(x)), x.flatten()]).T
# Convert y from 1d to 2d array.
y = y.reshape(100, 1)
# Number of Iterations for Gradient Descent
num_iter = 1000
# Learning Rate
alpha = 0.01
# Number of Training samples.
m = len(x)
# Initializing Theta.
theta = np.zeros((2, 1),dtype = float)
# Batch-Gradient Descent.
start_time = time.time()
for i in range(num_iter):
gradients = X_New.T.dot(X_New.dot(theta)- y)
theta = theta - (1/m) * alpha * gradients
# Print the model parameters.
print('model parameters:',theta,sep = '\n')
# Print Time Take for Gradient Descent to Run.
print('Time Taken For Gradient Descent in Sec:',time.time() - start_time)
# Hypothesis.
h = X_New.dot(theta) # Prediction on training data itself.
# Plot the Output.
plt.scatter(x, y, c = 'red')
plt.plot(x ,h)
plt.xlabel('Feature')
plt.ylabel('Target_Variable')
plt.title('Output')
输出:
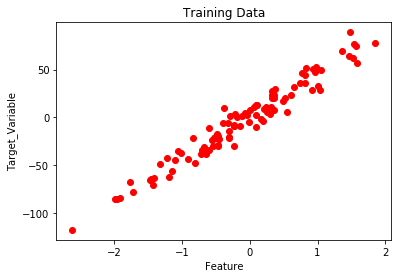
model parameters:
[[ 1.15857049]
[44.42210912]]
Time Taken For Gradient Descent in Sec: 0.019551515579223633
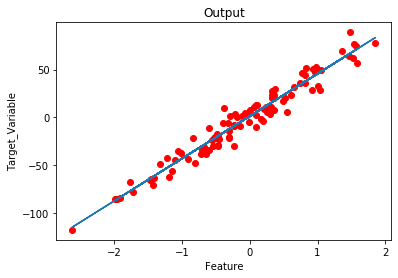
观察:
- 实施矢量化方法减少了执行梯度下降(高效代码)所需的时间。
- 易于调试。