R 编程中的比例 Z 检验
一比例 Z 检验用于在只有两个类别时将观察到的比例与理论比例进行比较。例如,我们有一群老鼠,其中一半是雄性,一半是雌性(p = 0.5 = 50%)。其中一些小鼠 (n = 160) 已患上自发性癌症,其中包括 95 只雄性和 65 只雌性。我们想知道,癌症是否影响男性多于女性?所以在这个问题中:
- 成功人数(男性患癌)为 95
- 观察到的男性比例 (p o ) 为 95/160
- 观察到的女性比例 (q) 为 1 – p o
- 男性的预期比例 (p e ) 为 0.5 (50%)
- 观察次数 (n) 为 160
比例 Z 检验的公式
检验统计量(也称为 z 检验)可以计算如下:
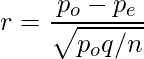
where,
po: the observed proportion
q: 1 – po
pe: the expected proportion
n: the sample size
R中的实现
在 R 语言中,用于执行 z 测试的函数是binom.test()
和prop.test()
。
Syntax:
binom.test(x, n, p = 0.5, alternative = “two.sided”)
prop.test(x, n, p = NULL, alternative = “two.sided”, correct = TRUE)
Parameters:
x = number of successes and failures in data set.
n = size of data set.
p = probabilities of success. It must be in the range of 0 to 1.
alternative = a character string specifying the alternative hypothesis.
correct = a logical indicating whether Yates’ continuity correction should be applied where possible.
示例 1:
假设 70 人中有 30 人向他们的朋友推荐 Street Food。为了检验这一说法,随机抽取了 150 人样本。在这 150 人中,有 80 人表示他们向朋友推荐 Street Food。这种说法准确吗?使用 alpha = 0.05。
解决方案:
现在给定 x=.80,P=.30,n=150。我们想知道,人们是否向他们的朋友推荐街头食品而不是健康食品?让我们在 R 中使用函数prop.test()
。
# Using prop.test()
prop.test(x = 80, n = 150, p = 0.3,
correct = FALSE)
输出:
1-sample proportions test without continuity correction
data: 80 out of 150, null probability 0.3
X-squared = 38.889, df = 1, p-value = 4.489e-10
alternative hypothesis: true p is not equal to 0.3
95 percent confidence interval:
0.4536625 0.6113395
sample estimates:
p
0.5333333
- 它返回 p 值,即 4.186269
- 替代假设。
- a 95% 的置信区间。
- 成功概率为 0.53
检验的 p 值为 4.486269,小于显着性水平 alpha = 0.05。 70 人中有 30 人向朋友推荐街头美食的说法并不准确。
示例 2:
假设目前的维生素药丸治愈了 80% 的病例。一种新的维生素丸被发现或制成。在接受新维生素治疗的 150 名缺乏维生素的患者样本中,有 95 人治愈。这项研究的结果是否支持新维生素比现有维生素具有更高治愈率的说法?
解决方案:
现在给定 x=.95,P=.80,n=160。让我们在 R 中使用函数prop.test()
。
# Using prop.test()
prop.test(x = 95, n = 160, p = 0.8,
correct = FALSE)
输出:
1-sample proportions test without continuity correction
data: 95 out of 160, null probability 0.8
X-squared = 42.539, df = 1, p-value = 6 . 928e-11
alternative hypothesis: true p is not equal to 0.8
95 percent confidence interval:
0.5163169 0.6667870
sample estimates:
p
0.59375
- 它返回 p 值,即 6.928462
- 备择假设
- a 95% 的置信区间。
- 成功概率为 0.59
检验的 p 值为 6.928462,大于显着性水平 alpha = 0.05。用新维生素治愈的 160 人中有 95 人的说法是准确的。
示例 3:
假设在印度 15% 的人喜欢在家工作。在 100 人的样本中,喜欢在家工作的人有 25 人。这个说法准确吗?
解决方案:
现在给定 x=.25,P=.15,n=100。让我们在 R 中使用函数binom.test()
。
# Using binom.test()
binom.test(x =25, n = 100, p = 0.15)
输出:
Exact binomial test
data: 25 and 100
number of successes = 25, number of trials = 100, p-value = 0.007633
alternative hypothesis: true probability of success is not equal to 0.15
95 percent confidence interval:
0.1687797 0.3465525
sample estimates:
probability of success
0.25
- 它返回 p 值为 0.007633
- 替代假设。
- a 95% 的置信区间。
- 成功概率为 0.25
检验的 p 值为 0.007633,小于显着性水平 alpha = 0.05。 100 人中有 25 人喜欢在家工作的说法并不准确。